Predicting Word Learning from Infants’ Eye-Tracking Signals
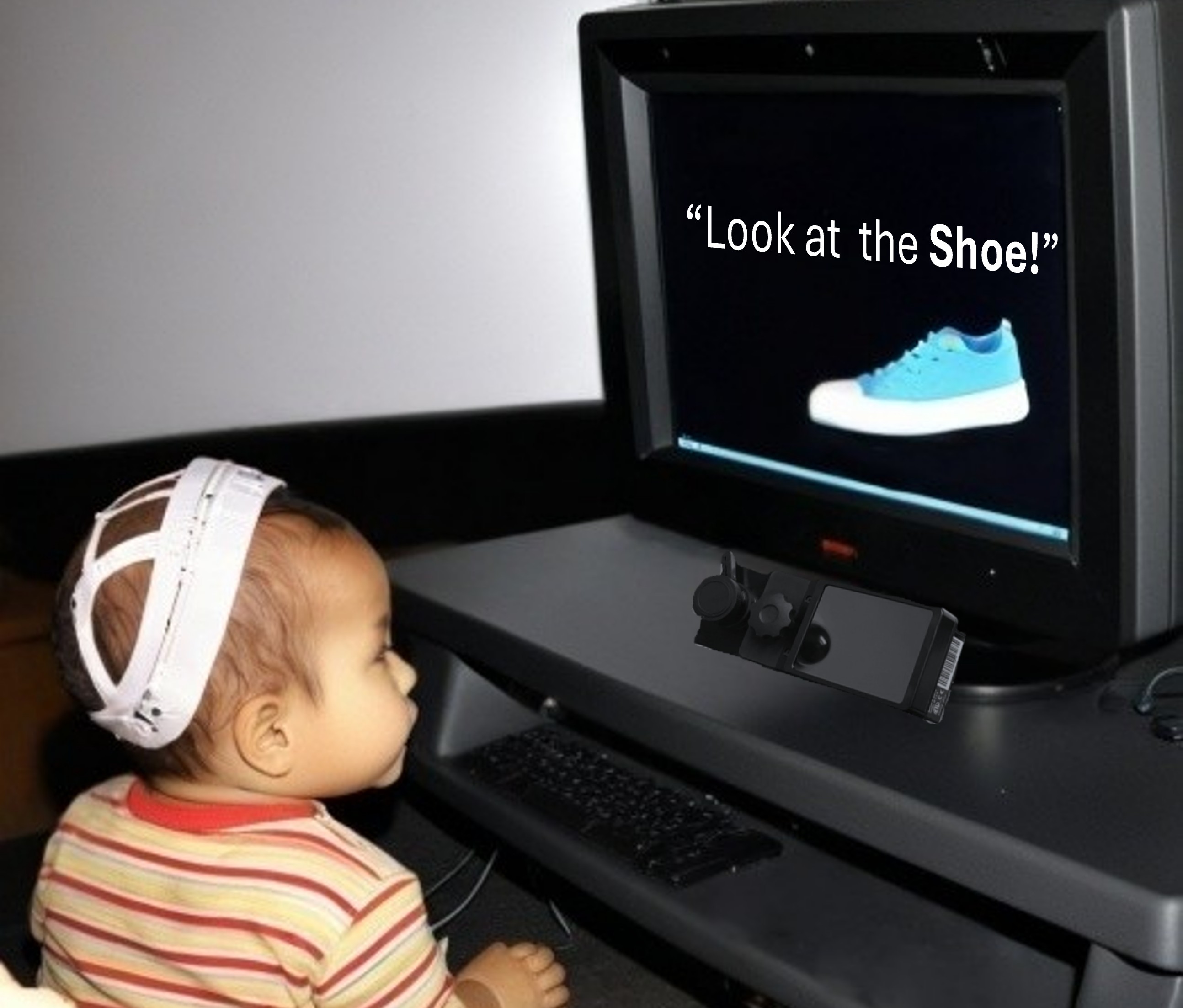
Drs. Janet Werker (Psychology) and Cristina Conati (Computer Science) have been awarded the DSI Postdoctoral Matching Fund for their project titled "Predicting Word Learning from Infants' Eye-Tracking Signals".
Summary
While research has shown which features of early social interactions may facilitate word learning in infancy, a mechanistic understanding of this is still missing. We propose one such mechanism: specifically that temporal regularity in infant-directed communication engages infants’ cognitive processes and underlying neural activity to facilitate word learning. This study will thus test whether temporal regularity in infant-directed speech facilitates word learning by means of engaging infants’ visual processing, and whether this is anchored by underlying neural processes. We will develop machine learning algorithms to predict word learning outcomes using infants’ eye-tracking data. We will also explore whether additional information from simultaneously recorded neural (EEG) and affective (facial expression) signals can improve our models.
Background
Early word learning occurs through social interactions where infants are presented with a plethora of cues that engage their visual, affective, and neural processesbut these have typically been studied separately. Additionally, researchshows that temporal regularity inherent to infant-directed communication engages infant attention and increases their neural tracking of the speech rhythm. However, exactly how regularity in ID communication systematically engages infants’ online processing (indexed via eye-tracking), and/or neural processes (explored in future analyses) to facilitate word learning is unknown. Machine learning techniques can help address these questions and allow consideration of more types of data simultaneously. To our knowledge, this will be the first work to employ ML techniques to predict learning outcomes in infants.
Challenge
The coarse measures typically used in infant learning studies, such as average looking times over long durations, obscure the actual learning process. Machine learning (ML) methods can increase the granularity and efficiency of quantifying infant learning, allowing us to better test our hypothesis that word learning will be better under temporally regular compared to irregular contexts. This project will thus be the first to employ ML techniques to extract features from infants’ eye-tracking data that predict learning outcomes. Further analyses will additionally explore whether our ML models improve with the inclusion of infants’ neural (electroencephalography; EEG) and/or affective (facial expressions) signals.
Research Goals
This research will first design and carry out a research study where we collect data from at least 30 infants, and then the main goal will be to develop ML techniques to determine if infant word learning benefits from temporal regularity. Specifically, we aim to predict infant learning outcomes from eye-tracking data. A secondary goal of the project will be to explore whether adding infant neural tracking data (measured by EEG), and affective state (indexed by their facial expressions), may help improve our models.