Leveraging data science to measure educational equity in Canadian post-secondary science
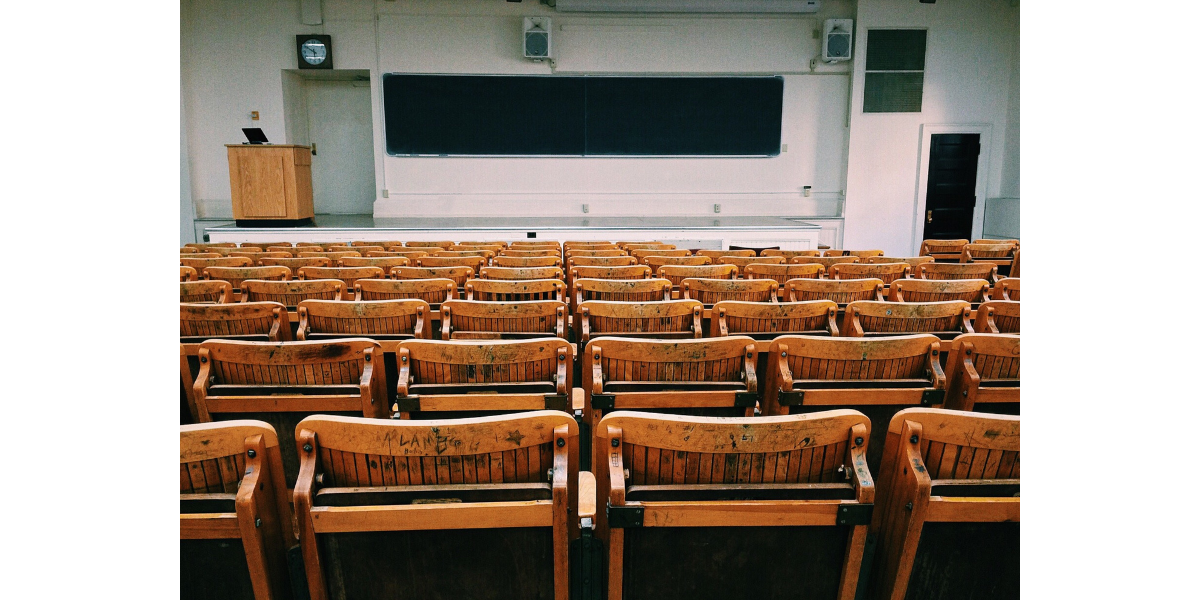
The Data Science Institute is pleased to announce a research team led by Drs. Joss Ives (Physics & Astronomy) and Jackie Stewart (Chemistry) has been awarded the DSI Postdoctoral Matching Fund. The multi-institutional research applies critical research methodologies to identify systemic and structural barriers to achievement, and to identify relationships between the instructor-created classroom climate and students’ learning, sense of belonging and persistence in STEM. The data set is evolving and complex, with data science challenges that include low proportional representation of many groups within the data set and complicated analysis variables, such as multiple-selection racial-ethnic identity. The team will use their models and data visualizations to inform educators across Canada about the extent to which their courses are equitable.
Background
The Canadian Consortium of Science Equity Scholars (CCSES) is a new group of educators and researchers dedicated to enhancing equity in post-secondary science courses. CCSES is collecting data in science courses at UBC, the University of Calgary, the University of Toronto, York University, and other institutions to investigate and improve equity in university-level science courses to better support all students, especially those who are historically or currently underrepresented. By examining disaggregated sociodemographic data, CCSES has pinpointed evidence of inequities of achievement that lead to underrepresentation in various STEM fields.
Challenge
The group's model of continuous, ongoing and expanding data collection across multiple courses and institutions will allow them to generate data sets of sufficient size to overcome some of the statistical limitations associated with populations with very low proportional representation. For example, their gender identity variable has woman, man, and nonbinary, and the size of the nonbinary group has previously been too small to draw meaningful conclusions. However, with a sufficiently large data set the team will be able to include nonbinary gender in our equity models in a meaningful way and will pursue effective methods of visualizing these data. Additionally, the team anticipates that their data sets will be sufficiently large to allow them to drill down and apply their modeling and visualization techniques to intersectional identities, which is an aspect of this type of research that is limited severely by low proportional representation.
Research Goals
Developing a better understanding of when sample sizes become large enough to include low-proportion groups in the statistical models, including those that result from multiple-selection identity questions, and how best to represent inequities visually.