Application of untrained machine learning analysis of multivariate sediment provenance data to critical metals exploration
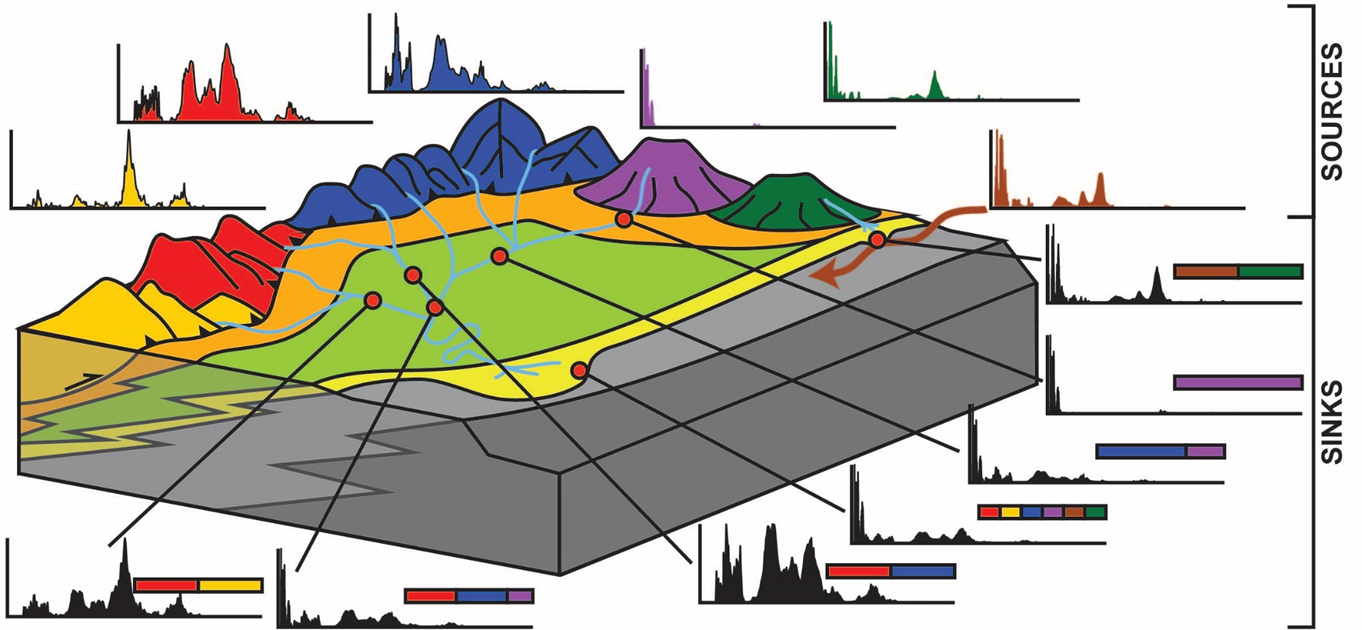
Drs. Joel E. Saylor (EOAS) and Michael Friedlander (Computer Science) have been awarded the DSI Postdoctoral Matching Fund for their project "Application of untrained machine learning analysis of multivariate sediment provenance data to critical metals exploration".
Summary
As major sources of copper (Cu) have been discovered, there is a critical need to identify smaller, more remote, or buried Cu sources to accommodate the increased proportion of the electricity that comprises the power supply. Geochemical features of zircon minerals from river sediment may be used to identify whether the igneous rocks from which they are derived contain Cu and hence whether the catchment is worth further exploration. Exploitation of this data requires a multi-variate approach capable of identifying unique endmembers in a mixed data set and assign proportions of the mixed data set to each of the endmembers. This project will test a method that we have developed to identify endmembers from zircon data sets.
Background
Prior research which targeted zircons from bedrock rather than sediments identified unique geochemical signals of Cu fertility. These geochemical signals should be retained when the zircons are eroded and transported downstream. However, some of the geochemical signals have been shown to covary with parameters unrelated to Cu fertility such as crustal thickness. Nevertheless, recent research indicates that a multi-variate approach to zircon geochemistry is likely to be successful at discriminating fertile versus barren porphyry bodies where an approach using a single geochemical proxy would fail.
Challenge
There are several data science problems to be addressed. The numerical multivariate unmixing model was developed using a numerical data set. Hence, the first challenge is assessing the current model on natural data sets which incorporate significantly more complexity than the artificially generated toy data set with on which the method has been tested to date. The second is to determine if we can discriminate Cu fertility from the other signatures in the zircon geochemistry data. Lastly, the research team will test the new algorithm with empirical data sets from the same South American rivers used for the initial test in order to assess whether the refined method leads to refinement of the data interpretation.
Research Goals
The research team will assess whether the proportion of zircons with geochemical indicators of Cu fertility will increase with increased proximity to active mining or known ore bodies. The team will further assess whether there is a spatial trend in the proportion of Cu-fertile zircon indicators in rivers with no Cu porphyries in their catchment. This will be further validated by comparing the proportions from the unmixing model to upstream areas occupied by geological formations which may act as sediment sources. The predicted increase in the proportion of Cu fertile indicators provides a basis to assess our estimation of the confidence level based on detrital indicators. The approach taken here will allow the research team to scale their observations and conclusions from the regional (1000s km2 catchments) to the local (10s km2 catchments) and answer the question, “How close to the deposit do you need to get before you start to see Cu-porphyry detritus, and how many grains should you analyze?”