Technology pipeline for the development of Machine-Learned Interatomic Potentials
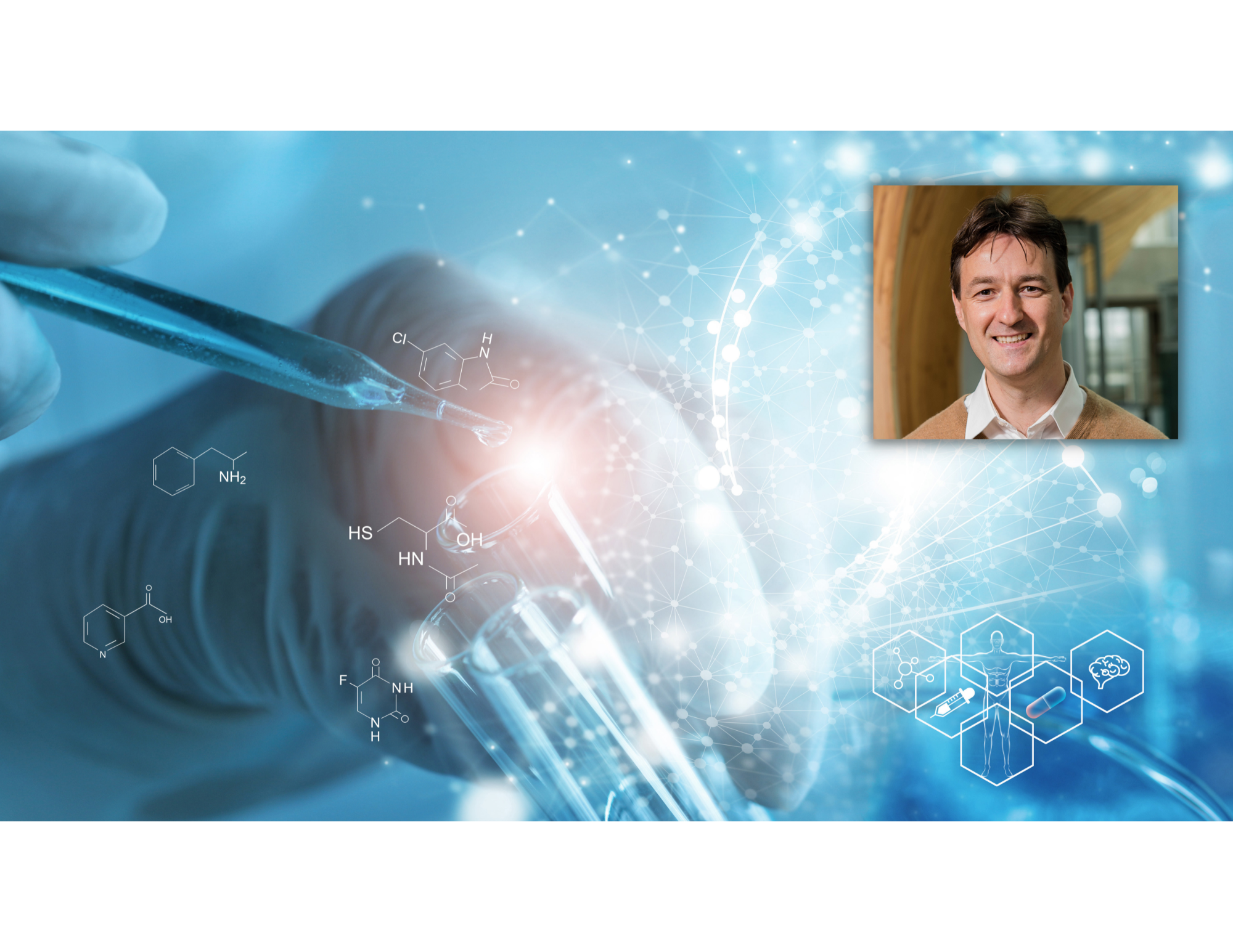
With the postdoctoral funding award from the Data Science Institute, Dr. Joerg Gsponer (Biochemistry and Molecular Biology) aims to establish and benchmark a technology pipeline for the development of Machine-Learned Interatomic Potentials (MLIPs) for Intrinsically Disordered Proteins (IDPs), thereby establishing a pathway to close a huge methodology gap that currently prevents significant progress in many areas of biochemistry and biomedicine.
Summary: Cellular communication and decision making relies primarily on proteins; their ability to catalyze chemical reactions and interact with each other in a specific and tunable manner. Although immense technological innovations in the fields of biophysics and computer science over the last six decades have provided unprecedented insight into protein structure and function, there still remains the under explored and under characterized “dark matter” of the protein universe: Intrinsically disordered proteins (IDPs) are central integrators and decision makers in cellular systems because their lack of a single structure lets them act like Swiss army knives with multiple functional “tools” that can be deployed as most appropriate for cellular challenges. However, the fact that they are dynamic, interconverting structural ensembles has hampered their characterization. Even molecular dynamics simulations fail to accurately capture IDP ensembles because the available empirical force fields have been developed and optimized to reproduce dynamics of folded proteins. The recent combination of machine-learning and data science technology has led to the development of machine-learned interatomic potentials (MLIPs). MLIPs provide a realistic route to revolutionize the field of IDP characterization by enabling accurate, large-scale atomistic simulations and modeling. This project aims to establish and benchmark a technology pipeline for the development of MLIPs for IDPs, thereby establishing a pathway to close a huge methodology gap that currently prevents significant progress in many areas of biochemistry and biomedicine.
Background: Research effects across the biomedical field try to elucidate how IDPs regulate cellular processes, how their vital contributions to cell physiology are affected in human disease, and how we can exploit IDPs as drug targets. However, the fact that their contributions to cell function rely on highly dynamic, often only transient, interactions with themselves or other molecules, which are nonetheless very specific, makes their characterization extremely challenging. A prime example for this challenge are IDPs that drive the assembly of cellular condensates via the process of phase separation. In these biomolecular condensates, IDPs contribute to a mesh of transient interactions that define the condensates’ visco-elastic properties and, thereby, their functional impact in a cell. Indeed, mutations in certain IDPs can lead to a “solidification” of IDP interactions in the condensates, resulting in a liquid-to solid transition and the formation of aggregates as found in neurodegenerative diseases like Alzheimer’s disease or ALS. Understanding and exploiting IDPs requires accurate molecular models capturing their highly dynamic nature.
Challenge: Empirical interatomic potential models have been instrumental in the emergence of large-scale molecular dynamics (MD) simulations as an indispensable tool in the characterization of complex protein systems that are highly dynamic. However, currently available models are only suitable for the characterization of folded proteins but not study the much larger phase space of IDPs. We require significantly better means to model molecular interactions between IDPs and a solvent (mainly water) and how these solvent interactions (or solvation) is affected by thermodynamic parameters (e.g. T, pH) or salt.
Research Goals: The overall goal of the project is to establish and benchmark a technology pipeline for the development of accurate, computationally efficient and robust MLIPs (force fields) for quantitative atomistic modeling of IDPs.