Gaussian Processes for Advancing Understanding of Planetary Magnetism with Spacecraft Observations
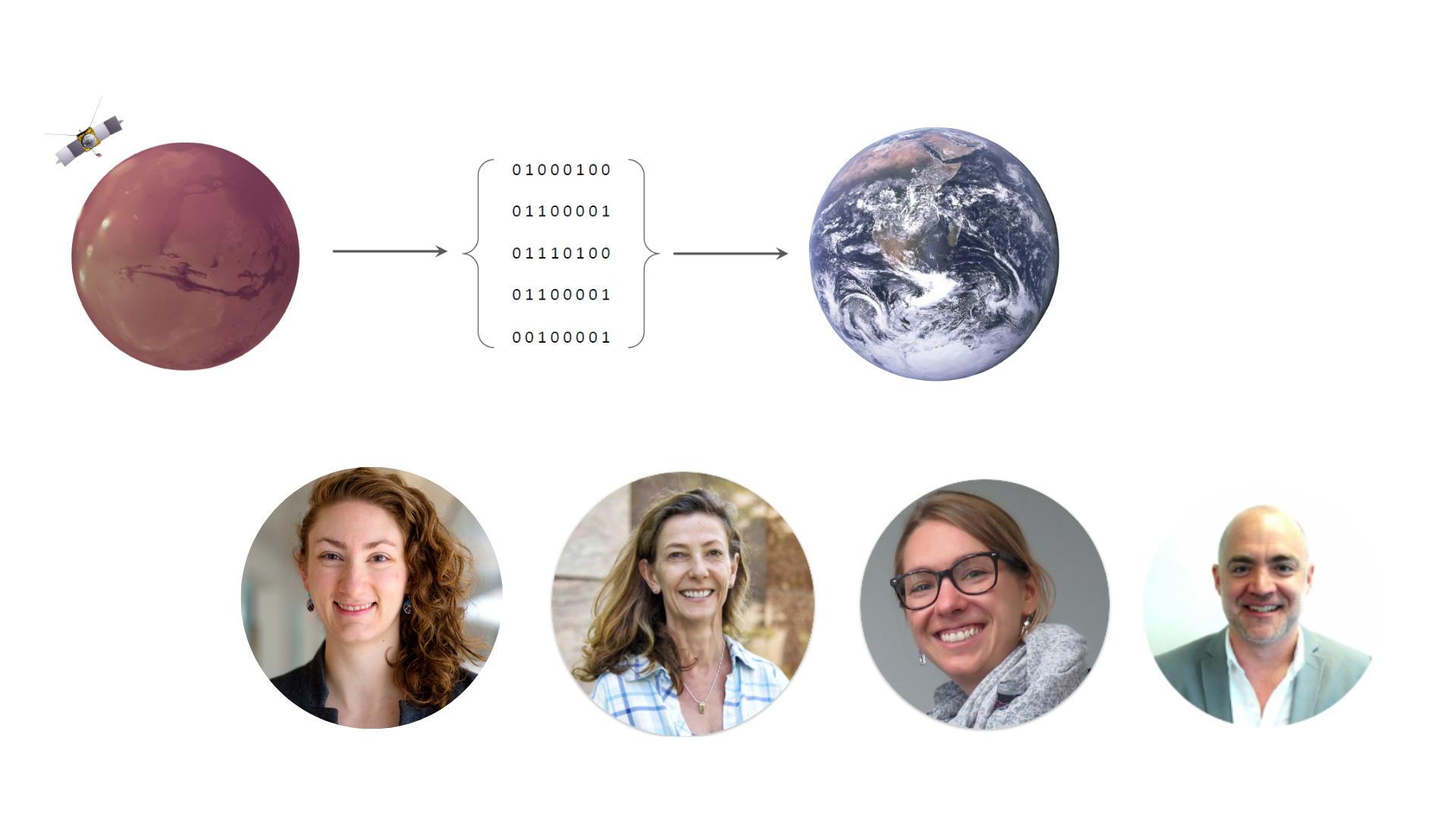
A research team, comprised of Dr. Abigail Azari with Drs. Catherine Johnson and Lindsey Heagy from the Department of Earth, Ocean, and Atmospheric Sciences, and Dr. Frank Wood from the Department of Computer Science, has been awarded the Post-Doctoral Matching Fund from the UBC Data Science Institute. This project aims to expand current development of Gaussian process-based estimations of the solar wind, focused on Mercury, Venus, and Mars, and develop integration of Gaussian processes into simulation-based inference, specifically focused on planetary magnetism.
Background: The characterization of a planet’s magnetic field is critical to advancing understanding on the planet’s formation, atmospheric and geologic processes, and planetary habitability. Spacecraft observations are the only direct source of data available to characterize planetary magnetic fields. Mercury, Venus, and Mars each have over a decade of spacecraft-obtained magnetic field observations, but these data are taken in irregular spatial distributions around the planet. Further complicating the use of these data is the natural variation of the solar wind, a supersonic flow of charged particles, that affects our observations of planetary data through physical processes which we are still understanding. Much of our current physics-based understanding of how the solar wind and planetary environments interact is represented through computationally large physics-based models. Full use of spacecraft magnetic field data to understand planetary magnetism then requires estimation and uncertainty quantification of the solar wind, and integration of these estimates into data analysis and physics-based models. Machine learning methods are the leading solution but uncertainty quantification and incorporation with physics-based models is challenging. This is an ongoing issue that has precluded many uses of machine learning in planetary science. It is an overarching goal of this project to address these challenges.
Challenge: Planetary science has seen half as many applications of machine learning methods as the field of Earth science (5.7% vs 11% of publications, see Azari et al. 2021). Planetary science, while sharing similar types of data as Earth science, doesn’t just benefit from, but requires methods that allow for spatiotemporal dependent uncertainty quantification, sparse datasets handling, and physical-model integration. This has limited the use the machine learning methods in planetary science and will continue to unless methods are provided with demonstrated applications..
Research Goals: The goals of this project are to provide solutions in uncertainty quantification, sparse dataset handling, and physical-model integration for planetary science. In this project we center on using Gaussian processes which enable elegant uncertainty quantification.