Anytime-Valid PAC-Bayes for Industrial Applications
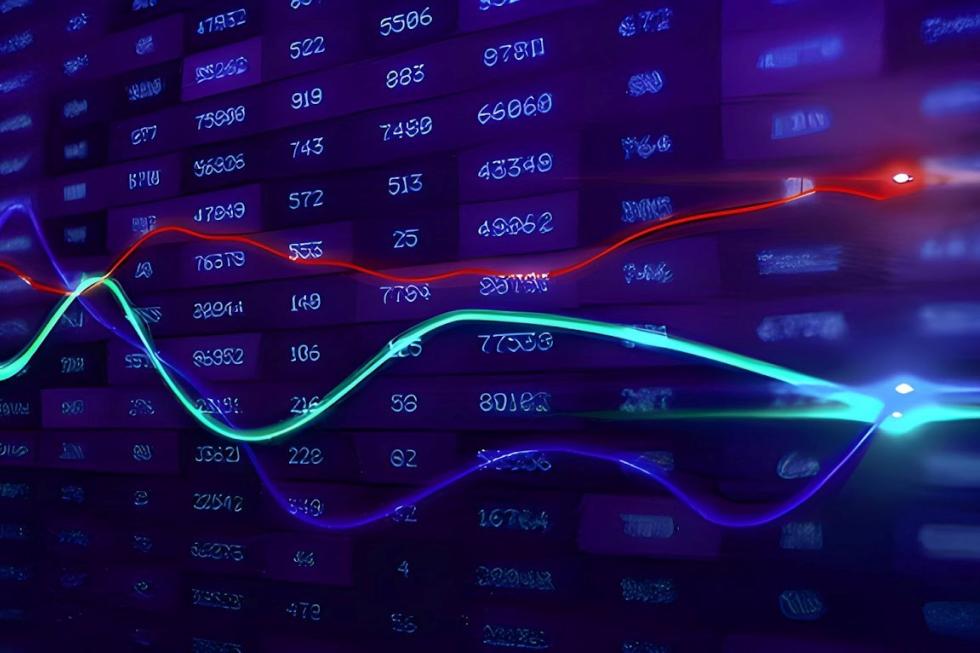
A research team led by Drs. Danica Sutherland (Computer Science) and Trevor Campbell (Statistics) were awarded postdoctoral funding from the UBC Data Science Institute. The team aims to provide a suite of tools which will allow companies to make optimal use of their data while providing rigorous, statistically principled and continously monitorable generalization guarantees on their deployed AI models.
Summary: Companies often need to track a variety of key business and performance metrics and take action if they change significantly. It’s also important, though, to avoid unnecessary action because of purely random fluctuations; the standard approach to identify which changes are “real” is statistical hypothesis testing. Traditional methods, however, are designed for “looking” only once; checking continuously breaks their guarantees on false positive rates. A growing number of companies – including Adobe, Microsoft, Amazon, and Netflix – have now adopted safe "anytime-valid" inference tools which remain valid when tested continuously. Currently, the anytime-valid tools deployed by these companies allow experimenters to, for instance, continuously monitor simple low-dimensional linear models which are amenable to well understood statistical tests. But companies are becoming increasingly interested in going beyond these simple models, and to incorporate recent breakthroughs in deep learning and AI into their products and analyses (e.g., object recognition in autonomous cars). There is thus growing need for an anytime-valid metric for generalization performance, which can be used as a decision criterion for determining when to retrain and redeploy sophisticated, non-linear, and high-dimensional models. In collaboration with researchers at Carnegie Mellon University, this project aims to extend the anytime-valid inference theory into the PAC-Bayes setting, with a focus on developing novel theory for concrete applications to be used in an industrial setting.
Background: Current approaches for assessing generalization performance are either vacuous when applied to the high-dimensional "foundation models" now ubiquitous in industry, or erode with each reuse of the held-out test set due to overfitting. Developing anytime-valid PAC Bayes bounds would allow organizations to, for instance, 1) continuously monitor the generalization performance of their models as new data becomes available, to decide when to retrain and redeploy; 2) select new data points to be labeled ("active learning") based on improvements to generalization performance; 3) control the amount of source data to be added into a given target domain ("domain adaptation") to improve model performance the target domain of interest; 4) prevent overfitting in deep models trained in a low-data regime, where a held-out validation set is unavailable; and 5) develop automated "stopping criteria" to determine when expensive data acquisition should terminate.
Challenge: Recent work from Aaditya Ramdas's group at Carnegie Mellon has established for the first time a general framework for developing anytime-valid PAC Bayes bounds, but significant work remains to develop algorithms and statistically grounded theory for particular industrial application areas. With the DSI’s support, the research team plans to develop novel theoretical advances and algorithms in the aforementioned research areas, and develop high-quality and easy-to-use open-source software packages for others to employ and extend.