Automating machine learning of interatomic potentials for green technologies
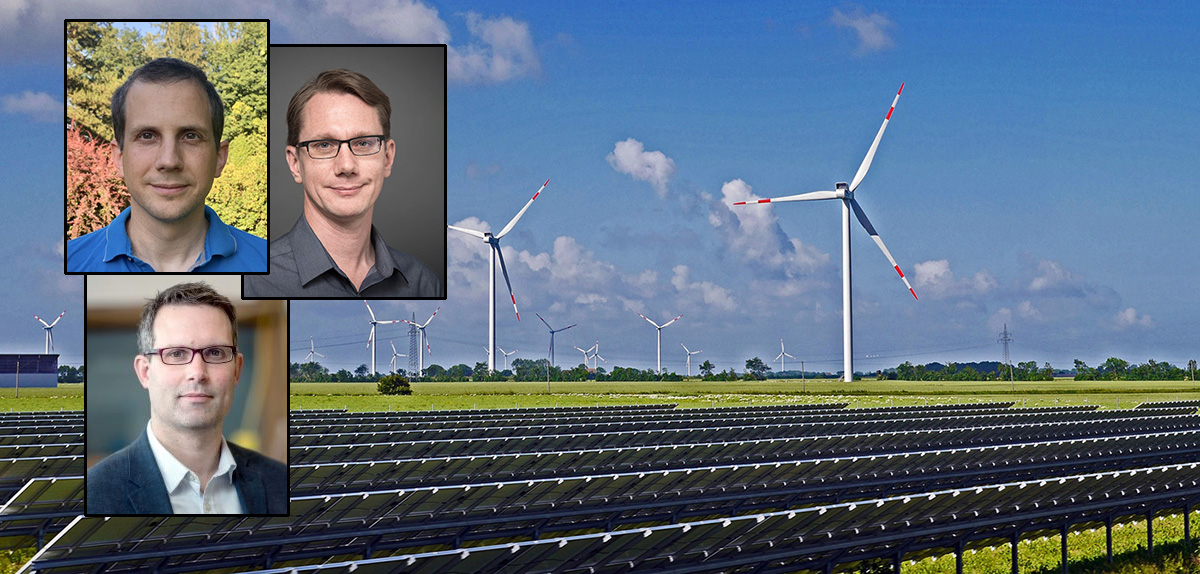
Christoph Ortner, Joerg Rottler, Chad Sinclair
A research team led by Drs. Christoph Ortner (Mathematics), Joerg Rottler (Physics), and Chad Sinclair (Materials Engineering) were awarded postdoctoral funding from the UBC Data Science Institute. This project will develop and standardize methodology to quickly generate new robust machine-learned potential models (MLPs) to accelerate the advancement of new sustainable technologies. The hope is that the methods developed will significantly reduce environmental and ecological risks by bringing green technologies to market quickly.
Summary: Machine learning approaches for the construction of interatomic potentials were first introduced in 2007 and 2010. Despite significant evolution in methodology, the development of robust machine-learned potential (MLP)models remains an intricate and time consuming process reserved for a small group of domain experts. As a result, their application remains largely limited to systems already treated by developers. In principle, a key advantage of MLPs over traditional empirical potentials is the possibility of rapidly and automatically generating custom, reactive, interatomic potentials. These can achieve high accuracy in specific use cases that would be challenging for empirical models. Examples include catalysis by established and emerging complex materials such as high-entropy oxides, complex surface interactions as in coatings or active materials for energy applications in batteries and fuel cells. For industrial applications, rapid turnaround between project conception and initial simulation results is necessary for the potential of MLPs to be realized. This project aims to develop and standardize methodology to allow for the rapid generation of new MLPs for interfacial systems as outlined above, thereby bridging the gap between emergent academic methods and practical applications. The focus on catalysis is directly motivated by the requirements of industry and government agencies to develop new sustainable technologies that drastically reduce environmental and ecological risks. A significant aspect of this project will therefore be to liaise with potential industry partners, investigate potential project partnerships and understand how the emergent MLP methodology can be best placed to aid industrial research.
Background: Quantum chemistry methods provide robust and transferable models of interaction between atoms (and electrons), but their extreme computational cost makes them unsuitable for modeling atomistic processes at large time and spatial scales. As a result, researchers have developed empirical interatomic potential models for many decades that enable the required large-scale simulations. However, this comes at the cost of poor accuracy, poor transferability and is challenging in the presence of significant chemical complexity. Bringing machine-learning and data science technology into this domain has led to the development of machine-learned interatomic potentials (MLPs), which provide the first realistic route to extend ab initio quantum chemistry approaches to genuinely large-scale atomistic materials simulation.
Challenge: The development of accurate and robust MLPs remains an intricate and time consuming process reserved for few domain experts. Their application has therefore remained limited to the academic domain. MLPs must provide accuracy, ease of use, transferability, and low computational cost, which is difficult to achieve in unison. Both scientific and industrial research require faster turnaround than is possible with current methodologies.
The overarching long-term aim of the project is an end-to-end theory, computational tools and protocols, that enable the development of accurate and robust MLPs for new atomistic modeling scenarios routinely, efficiently, and with minimal user intervention.