Quantifying the cascade effects of mining on terrestrial and aquatic ecosystems in the North American context
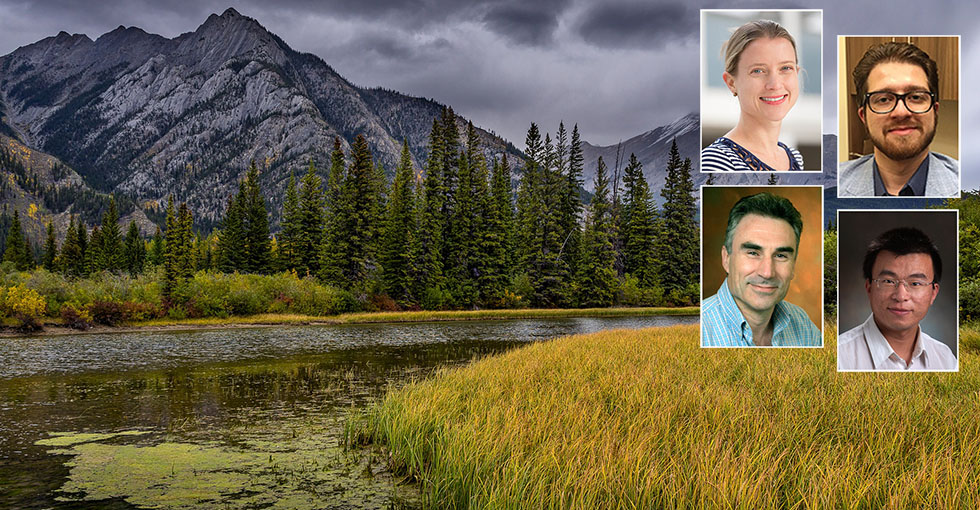
Nadja Kunz, Ali Ameli, Will Welch, Jiguo Cao
A team of UBC and SFU researchers led by Dr. Nadja Kunz was awarded DSI postdoctoral matching funds for their project that will examine the impacts of mining on the environment. Specifically, the team will build advanced statistical models using newly available data sets to better understand how mining is influencing hydrological variability, wildfires, and other environmental disturbances in Canada.
Summary: Human-made land developments can contribute to multi-dimensional environmental disturbances (e.g., wildfires and post-fire cascades of flood and drought cycles). The research team has been collaborating on research that combines hydrology and statistical science to investigate the hydrological causes and consequences of environmental disturbances, under a changing climate and land development, in key study regions within the North American context. This project will extend existing work by focusing on the influence of mining on hydrological variability and ultimately on multi-dimensional environmental disturbances. Additionally, it will contribute to ongoing research to investigate how future projections of climate change variability will influence hydrological cycles within key mining regions within the Canadian context.
Details: Current statistical models on causality in environmental sciences are typically univariate and/or stationary. These types of models are not able to consider the non-stationary multivariate relationships/feedbacks among landscape’s interrelated (and highly correlated) hydro-climatological and physical processes, and environmental disturbances. A large database of these features over many decades has been collected and is ready for model building. These will be combined with mainlining and geology datasets accessible through partnerships with the UBC Bradshaw Research Initiative on Mining and Metals (BRIMM). The focus of this research is to improve and apply nonstationary multivariate statistical frameworks, which can evolve through time and quantify these relationships/feedbacks. Specifically, the various types of time series are related to multivariate functional-data analysis (FDA). Most work on FDA has been typically for a single curve per observational unit as well as for stationary univariate outcome or covariate. Hydro-geo-climatic data, however, comprise multiple functional outcomes and covariates over time including functions for climatic variables, land cover extent and type, physical processes, and vulnerabilities. Quantification of the uncertainty of the complex relationships/feedbacks among functional outcomes and covariates is essential for environmental agencies to make robust water security assessment and land cover planning/management. In this regard, there are strong connections between FDA and Gaussian processes (GPs) to model complex functions. The proposed research program requires several extensions of FDA or GP methods. In essence, development of the statistical methodology in the proposed research program will combine and leverage the team’s expertise in FDA, time series and GPs.