Optimal placement of low-cost air quality sensors in Metro Vancouver to better predict air quality exposure
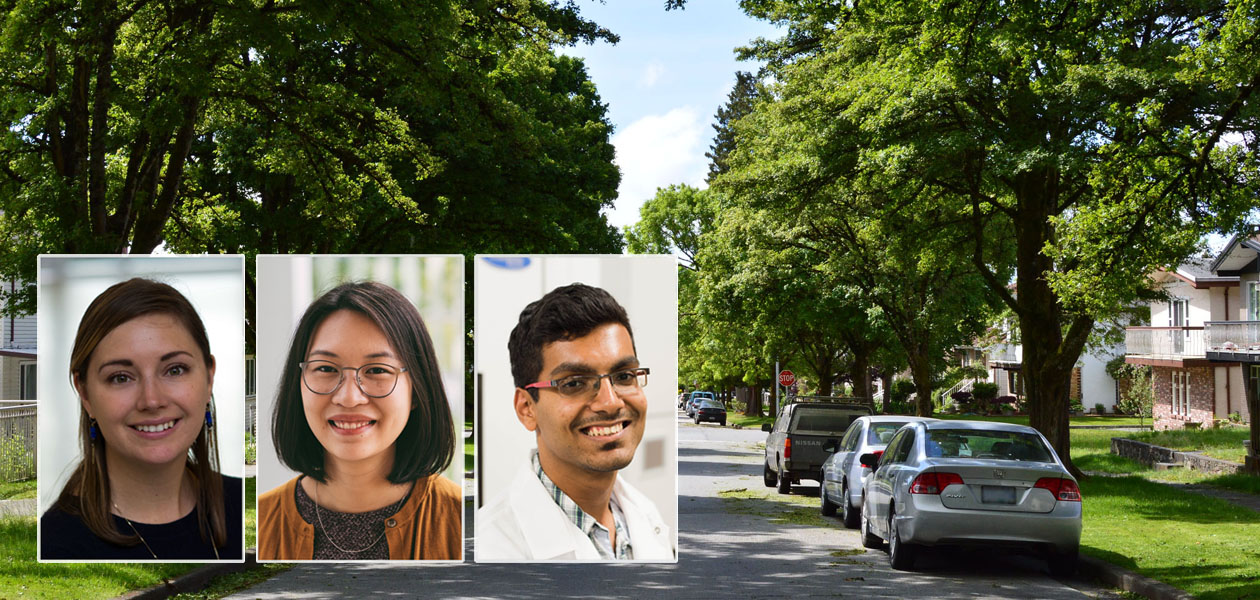
Drs. Naomi Zimmerman (UBC Mechanical Engineering) and Amanda Giang (UBC Institute for Resources, Environment & Sustainability) were awarded funding for their pilot project “Optimal placement of low-cost air quality sensors in Metro Vancouver to better predict air quality exposure”. This project seeks to develop efficient models for monitoring and predicting air quality in urban centres by leveraging anonymized 5G mobile location data with weather and air quality surveys. One key outcome is to identify locations for sensing of air quality—for example, what is the minimum number needed and where should they be installed for a given area. Ultimately the outcome is to enable data-driven public policy decision-making to support healthy outcomes as it relates to air pollution. This award will be supporting postdoctoral fellow Dr. Surya Dhulipala.
Summary: Air quality in cities varies both spatially and temporally. A limited number of existing fixed-site air quality monitoring stations fail to capture this variability. A dense network of low-cost air quality sensors can be used to create pollution maps, identify hotspots, drive public policy decision-making, and improve health outcomes attributable to air pollution. However, it is not possible to install and operate sensors in every neighborhood due to limited resources. It is also known that there is a risk of redundant information if sensors are not optimally placed. As part of this project, we will build an optimization model to determine the minimum number of sensors required to support air quality related decision-making while maximizing coverage area. Different use case scenarios will be tested including air pollution source identification/characterization and exposure estimation. Furthermore, we plan to use deep learning models to predict air quality in space and time. We will build and train the model using data from an existing low-cost sensor network (8 sensors) on UBC Vancouver campus for the period June 2021 – October 2021. During this period, we have access to other data sets through UBC’s partnership with Rogers Communication including traffic sensors, anonymized mobile phone data and air quality survey information. Finally, we will start building a large-scale air quality sensor network (~ 70 sensors) in Metro Vancouver using the results from our research. This step-by-step approach will enable us to dynamically update our models with real-world data completing a feedback loop and thus increasing accuracy of our models. Throughout this process, we will consult Metro Vancouver and focus on best value additions for their existing air quality monitoring network.
Details: There are three specific questions we would like to answer:
- How many low-cost air quality sensors are needed to accurately represent Metro Vancouver’s air quality on a hyper-local level?
- Where should these air quality sensors be placed?
- Can we predict air quality in space (where no sensors are installed) and time (e.g. 1 hour into the future)?
To answer these questions, we will use data collected using our low-cost sensors that can measure Particulate Matter (PM), Ozone, Nitrogen Oxides (NOx), CO and CO2, wind speed, wind direction, temperature, and relative humidity--in 15-second intervals. We are currently collecting air quality data at traffic intersections at UBC since June 2021. We also have access to other data sets through UBC’s partnership with Rogers Communication including traffic sensors, anonymized mobile phone data and air quality survey information. Publicly available data sets from scientific grade instruments operated by Metro Vancouver will be used in our project to help account for the variability of low-cost sensor data.
Our project objectives can be divided into two distinct data science problems:
- Number of Sensors & Sensor Placement Problem: We aim to minimize the number of sensors required while maximizing coverage area. This problem is commonly referred to as “Node Deployment Scheme” or “Location/Allocation Problem” in Operations Research. Models such as multi-objective optimization, Mixed Integer Linear Programming (MILP), Entropy-based semi-supervised learning (ESSL), and a combined Gaussian Processes (GP) + Krause’s Optimal Experimental Design (OED) algorithm will be used.
- Air Quality Prediction Problem: We will predict air quality in space (where no sensors are installed) and time (up to 1 hour in the future). Prospective techniques include machine learning models (Random Forest), artificial recurrent neural networks (Long Short-Term Memory) or an Agent-based approach.
Most of the above models are based on the sensing error of the deployed nodes and the estimation error of pollution concentration at locations where no sensor is deployed. It is also known that these models are highly sensitive to input parameters and that different models work better for different air pollutants. However, none of the previous studies, to our knowledge, have included factors such as meteorology, high variability of low-cost sensor data, traffic data sets and mobile phone data sets (within one framework).
In the first year, we plan to identify the appropriate objective functions and constraints. We also plan to develop a criterion for selection of sensor location. We will then evaluate different models used in literature and select appropriate models for answering the problem of sensor placement optimization and air quality prediction. By the end of the first year, we will begin installing air quality sensors across Metro Vancouver.