Blessings and curses of overparameterized learning: Optimization and generalization principles
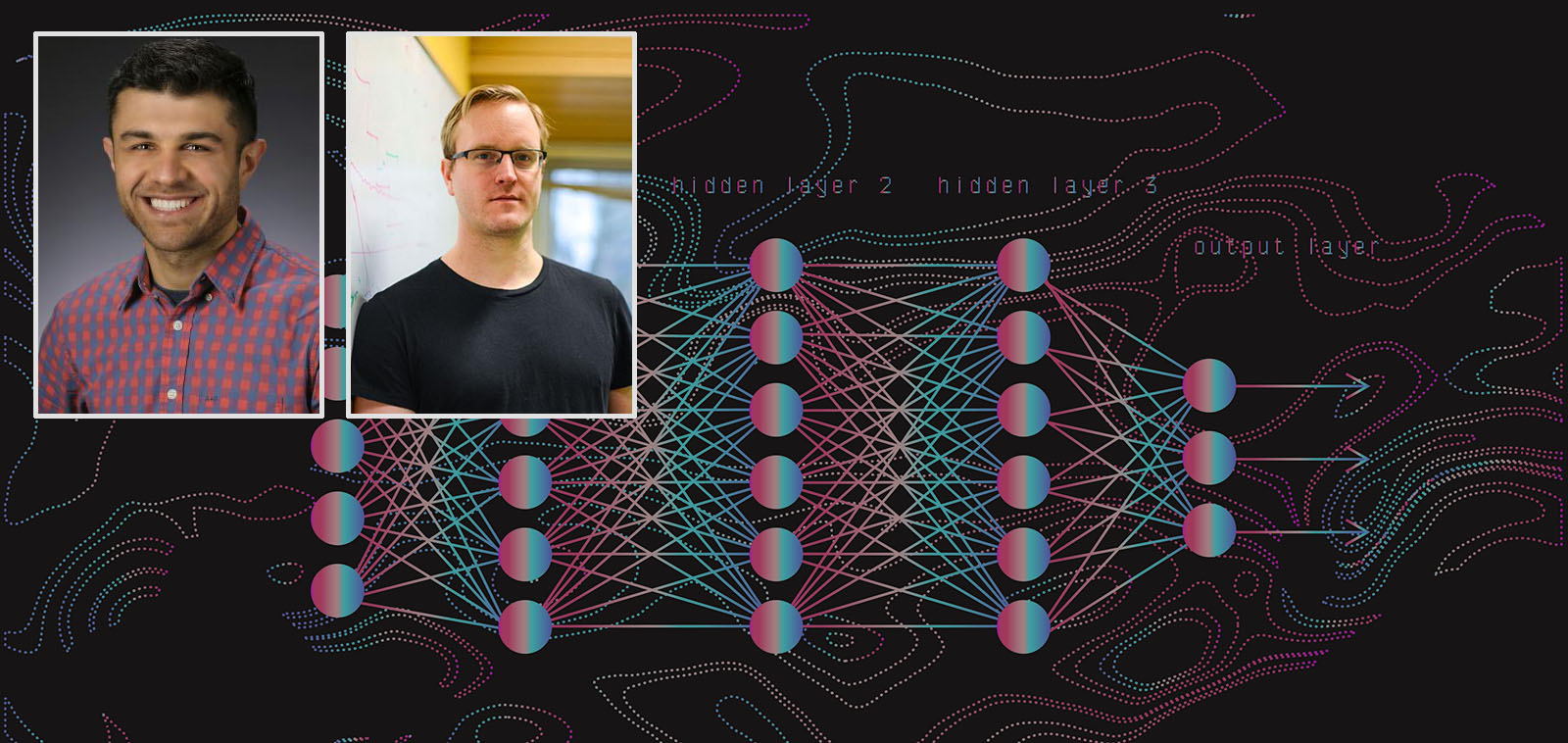
Drs. Christos Thrampoulidis and Mark Schmidt are teaming up to address unresolved challenges in the training of neural networks and its applications. With this postdoctoral funding award from the Data Science Institute, the team will combine their expertise in optimization and high-dimensional statistical learning theory to design more efficient training algorithms that are better suited for real-world use.
Summary: Deep neural networks are often exceedingly overparameterized and are often trained without explicit regularization. What are the principles behind their state-of-the-art performance? How do different optimization algorithms and learning schedules affect generalization? Does overparameterization speed up convergence? What is the role of the loss function on training dynamics and accuracy? How do the answers to these questions depend on the data distribution (eg. data imbalances)? This project aims to shed light on these questions by adapting a joint optimization and statistical viewpoint. Despite admirable progress over the past couple of years, existing theories are mostly limited to simplified data and architecture models (eg. binary classification, linear or linearized architectures, random feature models, etc.). The goal of the project is to extend the theory to more realistic architectures (eg. tangent-kernel regime, shallow and deep neural networks) and data models (eg. multiclass, imbalanced).
Details: This project will shine light on the fundamental statistical and optimization principles of modern machine-learning (ML) methods that aim to address system-design requirements on reliability, distributional robustness, safety, and resource efficiency. Such requirements become critical as we aspire to use data-driven ML algorithms to create automated decision rules in more aspects of everyday life. For example, in applications that directly involve data about people, such as decisions on who is granted a loan or who gets hired, we need to ensure fairness against demographic imbalances that exist in our society and translate to data. ML algorithms used for perception tasks in self-driving cars need to be safe against disturbances by adversaries. To effectively use modern deep-learning models – which are increasingly more complex, thus computationally expensive – in resource constrained platforms such as mobile devices, we need to carefully balance accuracy and resource efficiency.
Research Goals:
- Design efficient training algorithms and study their generalization for distributed overparameterized learning. We will extend the theory of implicit regularization to distributed settings. We are particularly interested in the effect of system constraints such as limited communication-bandwidth and limited memory constraints.
- Development of statistically grounded theory for training neural networks under data imbalances. In overparameterized learning, classical approaches to mitigate imbalances, such as weight normalization loss-adjustments, fail. Building on our recent works, we will propose alternative loss-adjustments and study the corresponding training dynamics and the induced generalization performance with respect to appropriate fairness metrics.
- Out-of-domain generalization remains a significant challenge for machine learning models. Approaches that aim to train invariant representations across domains are formulated in terms of non-convex optimization objectives whose optimization and generalization principles remain unexplored even in simple settings. We aim to understand the generalization principles of these methods building on appropriate extensions of our statistical models to account for domain shift. On the optimization front, we will investigate complexity lower bounds when using stochastic first-order methods. We also expect our study to have implications about the closely related problem of adversarial learning.