A deep learning approach to analyzing retinal imaging for medical diagnosis and prediction
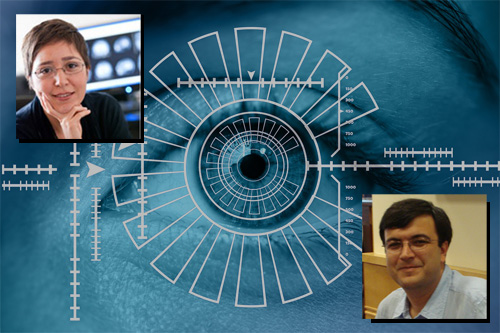
The DSI is excited to annouce that Drs. Ipek Oruc and Ozgur Yilmaz are awarded funding for their project on deep learning models for analyzing retinal images for medicial diagnosis and prediction. The funds from the DSI Postdoctoral Matching Fund Program will allow this research team to extend their work with deep neural networks to make predictions (i.e., classification) on various medical disorders ranging from neurological diseases to cancer using retinal images integrated with electronic medical records. One exciting highlight of their model is the ability to train neural networks to make accurate predictions from a relatively limited data set.
Deep learning (DL) techniques have seen tremendous interest in medical imaging, particularly via the use of convolutional neural networks (CNNs) for the development of automated diagnostic tools. The facility of their non-invasive acquisition makes various types of retinal imaging particularly amenable to such automated approaches. State-of-the-art tools include automated detection of clinical manifestations of ophthalmic diseases (e.g., visual features and lesions in the retinal image) as well as end-to-end blind classification of eye diseases.
Interestingly, recent work showed that traits thought not to be present or quantifiable in fundus images, such as patient age, sex, smoking status, and major adverse cardiac events can be extracted from fundus images using CNNs. Such work relies on access to massive datasets for training and validation, composed of hundreds of thousands of images. However, data residency and data privacy restrictions stymie the applicability of this approach in medical settings where patient confidentiality is a mandate. Our aims in this research project are two-fold: (1) “Go beyond eye diseases”: i.e. use DL techniques to diagnose and predict a wide variety of medical disorders from retinal fundus images; (2) “Use small data”: i.e. utilize generalization principles to achieve (1) with relatively modest-sized data sets.
Recently, our team showcased preliminary results for the performance of DL on small datasets to classify patient sex from fundus images. Specifically, we fine-tuned a Resnet-152 model whose last layer has been replaced by a fully-connected layer for two-class classification. We used stochastic gradient descent to train the model on 1500 retinal fundus images from 366 patients of known sex. We reported a validation error of 69.2% on the trained model. These results highlight usability and feasibility of DL methods when data is a limiting factor for automated analysis, and suggest a simple pipeline available to non-expert practitioners of DL.
While sex-classification on its own does not bear any clinical benefit, our work has provided a proof of concept—that traits invisible to the expert human eye, such as sex, can be extracted from fundus images using deep learning with relatively small datasets. We aim to extend this work to extraction of a wide variety of clinically-relevant classification questions, such as diagnosis and prediction of neurological (e.g., Alzheimer’s disease), cardiogenic (e.g., stroke) diseases, and malignancies (e.g., cancers of various types).