Robust, transferable and interpretable natural language processing of psychiatric clinical notes
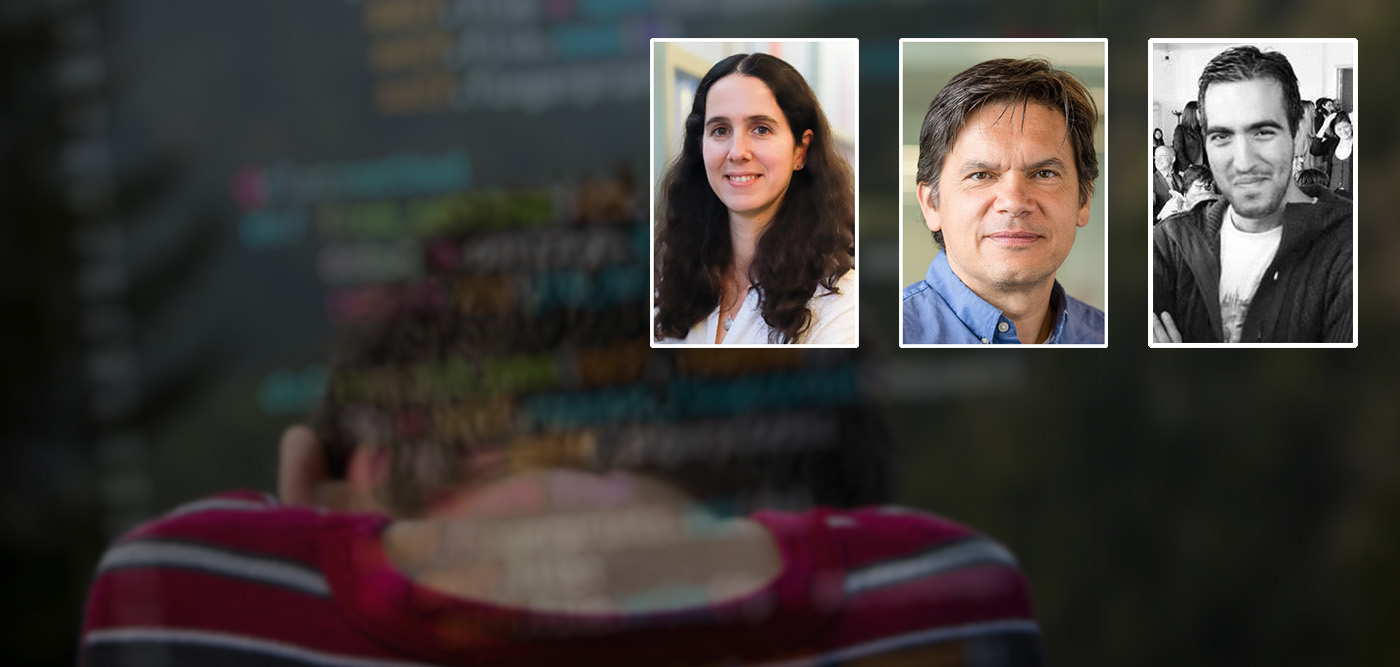
Elodie Portales-Casamar, Giuseppe Carenini
The UBC Data Science Institute is excited to fund a collaboration between Drs. Elodie Portales-Casamar (UBC Department of Pediatrics) and Giuseppe Carenini (UBC Computer Science) titled, "Robust, transferable and interpretable natural language processing of psychiatric clinical notes." The team includes postdoctoral fellow Dr. Ahmed Abura'ed, Dr. Ali Eslami (UBC Department of Psychiatry), and Ali Mussavi Rizi (PHSA). The project will implement the latest developments in natural language processing (NLP), such as the use of transformer models, to extract relevant information from clinical notes to support suicide prevention in at-risk children.
Summary: Suicide is the second-leading cause of death in adolescents in Canada. The Child and Adolescent Psychiatric Emergency (CAPE) unit at BC Children's Hospital (BCCH) provides stabilization and emergency intervention for youth in psychiatric crisis across BC and the Yukon. Approximately 45% of patients are admitted annually to CAPE for suicidality. Patients admitted to CAPE receive extensive diagnostic evaluation and assessment at admission and discharge, mostly recorded as free-text clinical notes which are difficult to incorporate in large-scale analyses. The primary aim of this project is to investigate whether NLP approaches can be successfully applied to CAPE clinical notes to extract clinically-relevant information and identify suicidality. Both traditional and neural approaches will be studied with a focus on interpretability and transferability of the resulting models.
Objective: Our primary objective will be to adapt and possibly extend state-of-the-art NLP techniques to CAPE admission notes, discharge summaries and other sources of structured information to
estimate suicide risk and other clinically-relevant information. The accuracy and interpretability of the resulting system will be thoroughly tested in several formative evaluations with domain experts in our team.
Details:
The specific questions the project will address:
- How can admission and discharge free-text clinical notes be leveraged to improve care in emergency interventions?
- How can we develop NLP analysis tools that are sufficiently accurate, as well as sufficiently interpretable by domain experts, to be used in clinical practice?
- How can these tools be incorporated in large-scale analyses, involving both textual and structured information?
- How can we build such tools so that they can be transferred across clinical domains?
Previously, we considered existing NLP tools directed at clinical notes. Leveraging insights from these efforts, we designed and prototyped our own Python-based NLP pipeline, with a special focus on exploring whether machine-learning based sentiment analysis could be informative in predict suicidality from psychiatric clinical. More specifically, we adapted the ABSApp tool to this task and published promising results.
The great success of deep learning in NLP recently has led to the development of large language models (i.e., large pretrained neural models such as BERT and GPT-3). These breakthroughs are enabling "transfer learning," whereby these state-of-the-art transformer models can be fine-tuned to complete a related but different task, such as sentiment analysis and text classification in NLP. In this project, we will further explore the benefits of sentiment analysis in suicidality prediction by investigating how these models can be effectively integrated with ABSApp. Initially, we will consider techniques in which aspect extraction and sentiment prediction are performed separately and sequentially. Then, we will study more sophisticated form of integration.
As a second goal in this project, we will explore how transformer models can be applied to predicting suicidality directly from clinical noted without any pre-processing (e.g., sentiment analysis) and compare with recent results on using weakly supervised neural models. The main challenge in deploying these deep learning models is that they suffer in term of interpretability. So, a second thread of this project will be to explore how to improve the interpretability of such models, which is critical in clinical settings. To this end, we will build on some initial progress we have recently made in improving accuracy and interpretability of text classifiers, especially on small datasets. Next, we will aim for an effective integration of those techniques with recent promising work on generating hierarchical explanations of neural text classification, which visualize how words and phrases are combined in their hierarchical structure (vs. a flat sequence) in determining the system predictions.